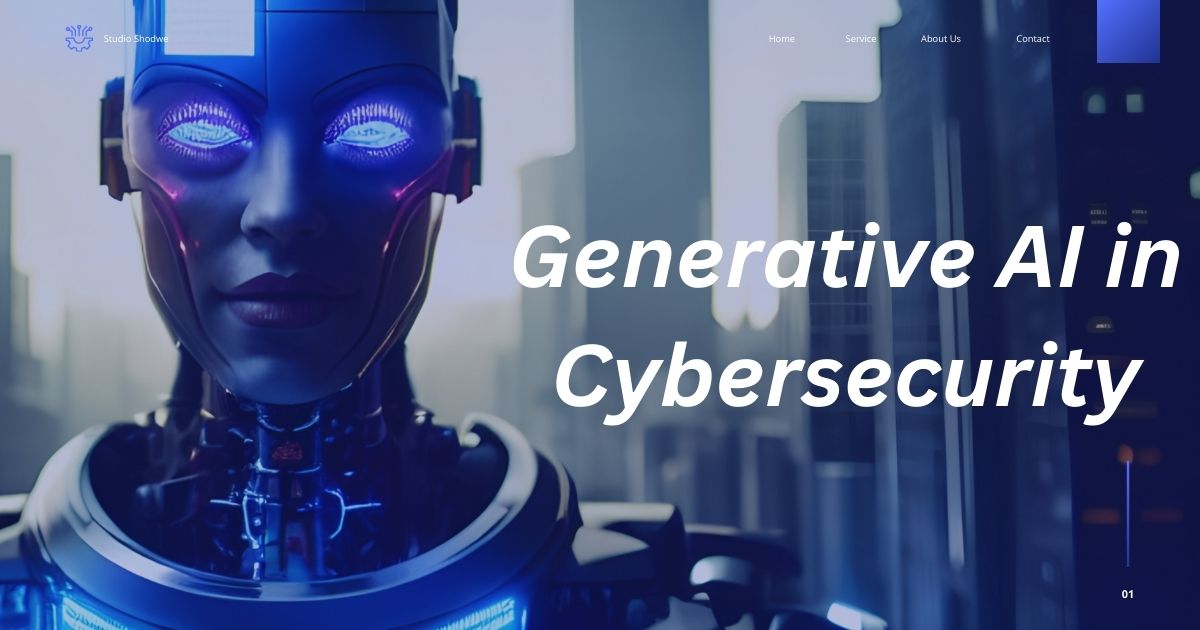
Introduction
What is Generative AI and Why is it Important in Cybersecurity?
Generative AI is a complicated branch of artificial intelligence which can create new statistics from contemporary information. In cybersecurity, it serves as a effective tool, producing synthetic records to simulate assault scenarios, model threats, and end up privy to vulnerabilities.
Unlike conventional AI, generative AI can actively create capability cyber threat fashions, assisting cybersecurity groups assume and reply to real-global dangers earlier than they increase. As cyber threats evolve, generative AI offers a sturdy, proactive method that enhances conventional security techniques, making it a vital useful resource for current cybersecurity.
How Cybersecurity is Adapting to Advanced Threats with AI
With the upward thrust in sophisticated cyber-attacks, traditional defense techniques regularly fall brief. Cybersecurity is evolving to fulfill the ones new demanding situations through leveraging AI, specially generative AI, to address complex, high-degree threats.
Generative AI permits protection agencies to apprehend and anticipate novel attack patterns, creating a protecting layer that evolves with growing threats. This adaptability is specifically essential for countering unknown malware traces, 0-day attacks, and AI-driven cybercrimes, marking a big shift in the direction of extra resilient, adaptive safety systems.
Key Takeaways
Major Benefits of Using Generative AI in Cybersecurity
Generative AI complements cybersecurity via imparting proactive danger detection, scalability, and actual-time efficiency. By simulating assault eventualities, it can help expect and prevent threats, making it less tough for organizations to live one step beforehand of attackers.
This generation permits stepped forward information assessment, taking into account a extra complete knowledge of vulnerabilities for the duration of big networks. The scalability of generative AI is right for organisations, in which handling big volumes of data and detecting real-time threats are crucial for a robust cybersecurity approach.
Potential Risks and Ethical Concerns of AI in Cybersecurity
While generative AI offers massive advantages, it moreover brings ethical dangers, in particular its functionality for misuse. Cybercriminals can use generative AI to create realistic phishing attacks, deepfakes, or synthetic identities, making fraud detection challenging.
Additionally, AI models can expand biases based totally absolutely on the statistics they’re professional on, probable overlooking effective threats.
These ethical and protection problems call for stringent rules, oversight, and apparent practices to balance the benefits of generative AI with accountable, managed utilization to keep away from unintended consequences.
Table 1: Overview of Generative AI Applications in Cybersecurity
Application Area | Role in Cybersecurity | Example Tool/Technique |
---|---|---|
Threat Detection | Identifies and neutralizes cyber threats early | Synthetic dataset generation |
Phishing Protection | Detects and blocks phishing attempts | Pattern recognition |
Intrusion Prevention | Creates traps to lure and study attackers | Honey trap or decoy systems |
Cybersecurity Training | Provides real-world attack simulation | AI-generated cyber threat models |
Malware Analysis | Analyzes and predicts new malware strains | Synthetic malware generation |
In-Depth Look: Generative AI Applications in Cybersecurity
Automated Threat Detection and Response
Generative AI can simulate an array of cyber-assault situations, enabling early detection and fast reaction. By creating artificial datasets of ability attacks, those models decorate a device’s ability to apprehend anomalies speedy, lowering reaction time substantially.
This proactive chance detection helps safety groups neutralize dangers earlier than they escalate. Automated response structures powered by means of generative AI analyze assault paths in real-time, constantly refining their technique to counter cyber threats with agility and accuracy.
Advanced Phishing and Malware Detection
Phishing and malware detection is considerably more suitable via generative AI, because it learns to become aware of diffused styles commonly related to these threats.
Generative AI models examine huge quantities of email and network data, figuring out markers that distinguish authentic communications from phishing tries. Similarly, via generating synthetic variations of acknowledged malware, those models can are expecting how malicious code might evolve, improving malware detection prices and ensuring structures are ready to address rising threats efficiently.
Tables of Comparison: Generative AI vs Traditional AI in Cybersecurity
Feature | Generative AI in Cybersecurity | Traditional AI in Cybersecurity |
---|---|---|
Data Handling | Uses synthetic data generation | Relies on historical or real-world data |
Proactive Threat Simulation | Simulates new and hypothetical threats | Focuses on known or historical threats |
Scalability and Efficiency | Highly scalable and adaptable | Effective but less flexible for complex attacks |
Common Challenges and Ethical Implications
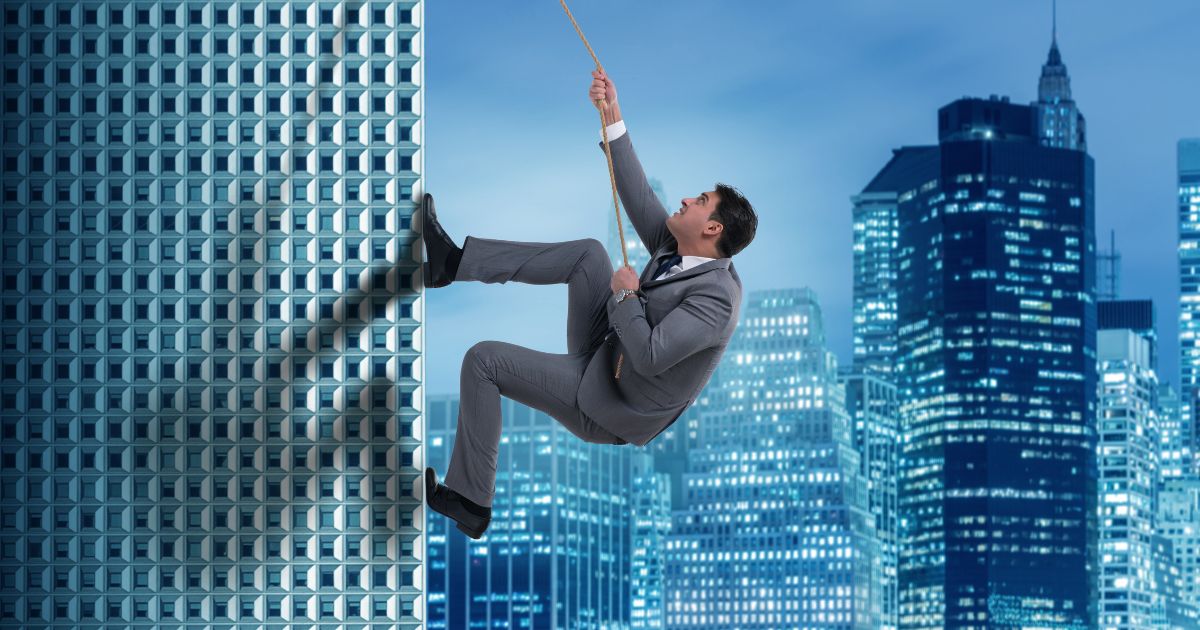
Technical Challenges in Integrating Generative AI with Cybersecurity Protocols
Integrating generative AI in cybersecurity is technically challenging, requiring specialized know-how and assets. AI fashions must be trained with a great dataset, ensuring they’re free from biases and capable of generalize effectively.
Additionally, maintaining these AI structures is costly and aid-intensive. Organizations should deal with these technical obstacles to leverage AI efficiently, investing in professional personnel and ultra-modern infrastructure to create a balanced, high-appearing security surroundings.
Ethical Implications of Generative AI in Security
Generative AI poses considerable ethical worries, as its misuse may want to permit cybercriminals to craft state-of-the-art assaults, inclusive of deepfakes and synthetic phishing content. The ability for AI-driven malicious programs demands stricter regulatory standards and ethical issues to prevent abuse.
Organizations and AI builders must prioritize accountable AI usage, implementing assessments and balances that make sure generative AI’s deployment aligns with ethical standards, aiming to defend, instead of jeopardize, consumer privacy and protection.
FAQs
How Does Generative AI Differ from Traditional AI in Cybersecurity?
Generative AI and traditional AI differ drastically in their technique to cybersecurity. Traditional AI commonly works with historic information, mastering from beyond styles to are expecting and manipulate threats.
In assessment, generative AI creates artificial information, simulating ability assault scenarios and novel threats that have no longer yet took place. This proactive first-rate lets in generative AI to count on and counter unknown cyber dangers, making it specially precious in managing rising and complex threats in cybersecurity.
Is Generative AI Reliable for All Cybersecurity Applications?
While generative AI is dependable in lots of cybersecurity packages, it isn’t foolproof. Its effectiveness depends at the exceptional of schooling statistics and the unique use case.
For instance, generative AI excels in hazard simulation and phishing detection but can also face challenges with nuanced, actual-time selection-making without human oversight.
Therefore, generative AI have to be seen as a powerful complement to cybersecurity, running great along conventional systems and professional human experts who can screen and manual its moves.
Conclusion
Future of Generative AI in Cybersecurity
Generative AI is about to come to be necessary to cybersecurity, offering structures with the intelligence to evolve alongside cyber threats. In the destiny, those fashions are expected to function greater autonomously, adapting to new varieties of assaults with out guide updates.
As AI technology progresses, generative AI will possibly integrate seamlessly into security protocols, enabling an adaptive, dynamic defense technique to maintain tempo with sophisticated cyber threats.
A Balanced Approach to Generative AI for Safer Digital Ecosystems
While generative AI offers transformative blessings, a balanced technique that includes regulatory tips, moral requirements, and human oversight is vital. By imposing responsible AI guidelines, agencies can leverage the strengths of generative AI even as minimizing ability dangers.
A balanced framework ensures generative AI’s contributions to cybersecurity stay powerful, moral, and aligned with the broader purpose of a secure digital environment for all customers.